What Is Responsible AI and Why Does It Matter in Data Science
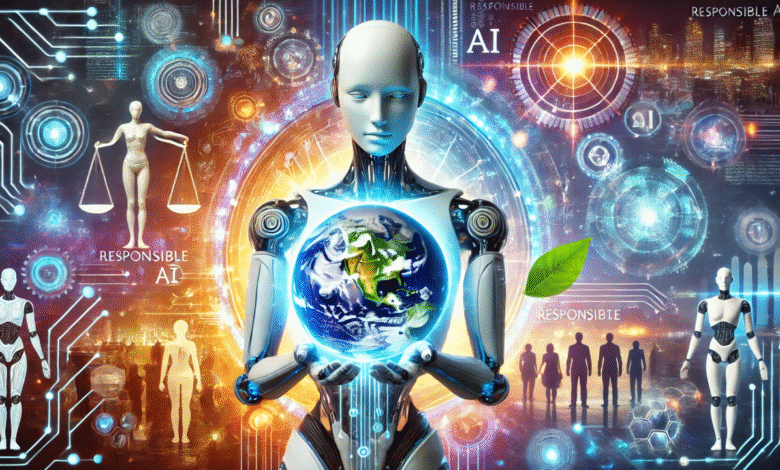
In today’s fast-moving tech world, Artificial Intelligence (AI) is transforming everything—from healthcare to finance to everyday apps we use. But with great power comes great responsibility. That’s where Responsible AI steps in. It’s not just a buzzword—it’s a crucial framework for building trustworthy, ethical, and fair AI systems. If you’re someone diving into the world of data science or AI, understanding responsible AI should be at the top of your list. (Explore this Data Science course if you’re getting started or looking to deepen your knowledge.)
What Exactly Is Responsible AI?
Responsible AI refers to the practice of developing and deploying AI systems in a way that aligns with ethical principles such as fairness, accountability, transparency, and privacy. In simple terms, it ensures that AI technologies do what they’re supposed to do—without causing unintended harm.
Imagine an AI hiring tool that favors one group over another due to biased data. Or a facial recognition system that performs poorly on certain ethnicities. These aren’t just technical issues—they’re real-world problems that affect people’s lives. Responsible AI helps prevent this by focusing on the impact of algorithms and data, not just their performance.
Why It Matters in Data Science
As a data scientist, you’re often at the heart of AI development. You select data, build models, and make decisions that can influence products, policies, and people. If the models you create are biased or opaque, they can erode trust or even reinforce discrimination.
Here’s why it matters:
- Bias Reduction: Responsible AI encourages you to assess data for bias before feeding it into models.
- Transparency: It promotes explainable AI, making sure stakeholders understand how a decision was made.
- Accountability: It helps assign responsibility if something goes wrong, so it’s clear whether it was a data issue, a model failure, or a deployment flaw.
- Privacy Protection: With regulations like GDPR, ensuring data privacy isn’t optional—it’s a legal requirement.
Real-World Examples
- Healthcare: AI models predicting disease risk must be fair across demographics. A biased model could lead to underdiagnosis in certain groups.
- Finance: In loan approval systems, Responsible AI helps ensure decisions aren’t biased against particular regions or genders.
- Social Media: Content recommendation engines need to be transparent and avoid promoting harmful content or echo chambers.
The Road Ahead
Responsible AI is not a one-time checkbox—it’s a continuous process. It requires collaboration between data scientists, ethicists, policymakers, and users. Companies like Google, Microsoft, and IBM have even created internal ethics boards to guide AI development. For individuals, learning how to integrate ethics into AI design is a skill that will only grow in value.
Whether you’re designing an algorithm or interpreting results, Responsible AI is your compass to ensure you’re building something that works for everyone, not just the majority or the most profitable.
So, if you’re thinking about building a career in AI or want to future-proof your skills, consider diving deeper into responsible and explainable AI practices. This Generative AI online course is a great way to explore how modern AI systems are created responsibly, especially as generative tools like ChatGPT and DALL·E become mainstream.