Colin Zhang Carlmont: A Trailblazer in Machine Learning and Drug Discovery
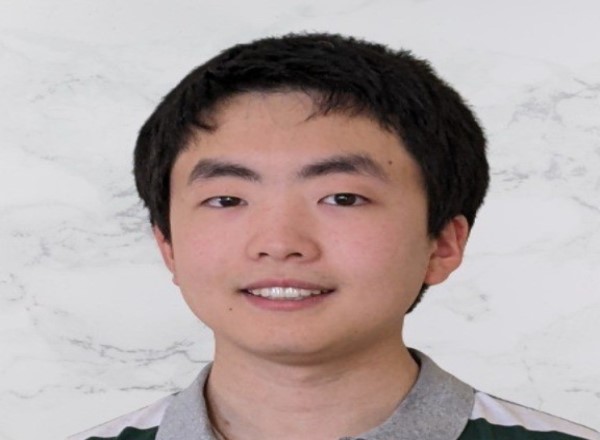
In the rapidly evolving fields of machine learning and drug discovery, Colin Zhang Carlmont is a name that stands out. As a young scientist from Carlmont High School, Colin Zhang has made significant strides in computational chemistry, machine learning, and artificial intelligence. His work, particularly in utilizing machine learning for molecular generation and drug solubility prediction, highlights not just his technical abilities but also his dedication to pushing the boundaries of modern science. This article delves into Colin’s impressive contributions, how they are shaping the future of drug discovery, and the broader implications of his work in scientific research.
Who is Colin Zhang?
Colin Zhang is a student at Carlmont High School who has gained recognition for his work in computational chemistry and artificial intelligence. His projects focus on the application of machine learning to drug discovery, specifically in the fields of molecular generation and solubility prediction. As an emerging young scientist, Zhang’s research has been published in esteemed platforms, gaining him visibility within the scientific community. His work not only highlights his passion for science but also showcases the potential of young minds to contribute to cutting-edge research.
The Role of Machine Learning in Drug Discovery
In the past decade, machine learning (ML) has revolutionized several industries, and drug discovery is no exception. Traditional methods of discovering new drugs are time-consuming and costly, often taking years or even decades before a new drug hits the market. However, with the advent of machine learning, researchers can now speed up this process by using algorithms that can analyze vast datasets, simulate chemical reactions, and predict molecular behaviors.
Colin Zhang, along with his collaborators, has been exploring how ML models, particularly autoencoders, can be utilized to streamline drug discovery. Autoencoders, a type of artificial neural network used for unsupervised learning, can help researchers discover new molecules by learning efficient data representations from large chemical datasets. This capability is crucial in the pharmaceutical industry, where identifying molecules with desired properties is often like finding a needle in a haystack.
Colin Zhang’s Contribution: Evaluating SMILES-based Autoencoders
One of Colin Zhang’s notable projects involves the use of SMILES-based generative autoencoders for molecular generation. SMILES (Simplified Molecular Input Line Entry System) is a notation that allows a user to represent a chemical structure in a way that a computer can understand. Zhang and his team trained these models for 200 epochs to investigate whether they could differentiate molecules based on key chemical properties such as molecular weight, partition coefficient, and hydrogen bond donors and acceptors.
Their results were groundbreaking. Zhang hypothesized that the autoencoder would preferentially encode molecular weight, a theory that was proven correct. The model showed a tendency to distinguish molecules primarily by their weight, with other chemical properties being considered to a lesser extent. Additionally, the generated molecules were strikingly similar to those in the training set, indicating that the model might be overfitting the data.
This discovery has significant implications for the development of machine learning models in drug discovery. Overfitting occurs when a model becomes too tailored to the training data and fails to generalize to new data, which is a challenge that researchers like Zhang are actively working to overcome.
The Challenges of SMILES-based Models
While SMILES-based autoencoders have shown promise in molecular generation, they are not without their challenges. One major limitation that Zhang identified in his research is that these models struggle to encode higher-level properties, such as molecular connectivity and structure. This limitation can hinder their usefulness in real-world applications, where the structure and connectivity of a molecule play a crucial role in its efficacy as a drug.
Zhang’s work suggests that while SMILES-based models are effective in encoding basic chemical properties, they may not be suitable for more complex tasks. His research is a step toward understanding the limitations of current machine learning models in drug discovery and is likely to inspire future studies aimed at mitigating these challenges.
Predicting Drug Solubility Using Machine Learning
In another project, Colin Zhang applied machine learning to predict the solubility of drug molecules. Solubility is a key factor in drug design, as it determines how well a drug can be absorbed by the body. Predicting solubility can help researchers identify drug candidates that are more likely to succeed in clinical trials, potentially saving years of research and billions of dollars.
Zhang’s research involved comparing two machine learning models: a linear regression model and a graph convolutional neural network (GCNN). While both models showed reasonably accurate predictions, the GCNN outperformed the linear regression model, particularly when dealing with larger datasets. This finding aligns with the general consensus in the machine learning community that neural networks tend to perform better with larger and more diverse datasets due to their ability to model complex relationships between data points.
Through this project, Zhang demonstrated that machine learning models can be highly effective tools in drug design, particularly in predicting properties like solubility. His work in this area has contributed to the growing body of evidence supporting the use of AI in pharmaceutical research.
Colin Zhang’s Vision for the Future of Drug Discovery
Colin Zhang’s research is not just about the present—it’s about the future of drug discovery. His work highlights the potential for machine learning to revolutionize the pharmaceutical industry by making drug development faster, cheaper, and more efficient. However, Zhang is also aware of the limitations of current technologies and is actively working to address these challenges.
One of the key issues he has identified is the need for better models that can generalize across different datasets. Overfitting remains a significant challenge in machine learning, and Zhang’s research suggests that more work is needed to develop models that can accurately predict chemical properties without relying too heavily on the training data.
Zhang’s vision for the future of drug discovery involves a combination of machine learning and traditional scientific methods. He believes that while machine learning has the potential to transform drug discovery, it should be used in conjunction with other techniques to ensure the best possible outcomes.
The Broader Impact of Colin Zhang’s Research
Colin Zhang’s research is not just limited to the academic realm—it has the potential to impact the pharmaceutical industry as a whole. By improving the efficiency of drug discovery, machine learning models like the ones Zhang is working on could help bring new drugs to market faster, potentially saving lives.
Moreover, Zhang’s work has broader implications for the field of artificial intelligence. His research demonstrates the power of AI in solving complex scientific problems and highlights the need for continued investment in this area. As machine learning continues to evolve, researchers like Zhang will play a crucial role in shaping its future applications.
Conclusion
Colin Zhang Carlmont is a name to watch in the fields of machine learning and drug discovery. His work on SMILES-based autoencoders and drug solubility prediction has the potential to transform the pharmaceutical industry by making drug development more efficient and cost-effective. However, his research also highlights the challenges that remain, particularly in the areas of model generalization and overfitting.
As Zhang continues his studies and expands his research, there is no doubt that he will continue to make significant contributions to the fields of computational chemistry and machine learning. His vision for the future of drug discovery, which combines cutting-edge technology with traditional scientific methods, is likely to inspire a new generation of researchers and innovators.
With young minds like Colin Zhang leading the charge, the future of drug discovery looks brighter than ever.